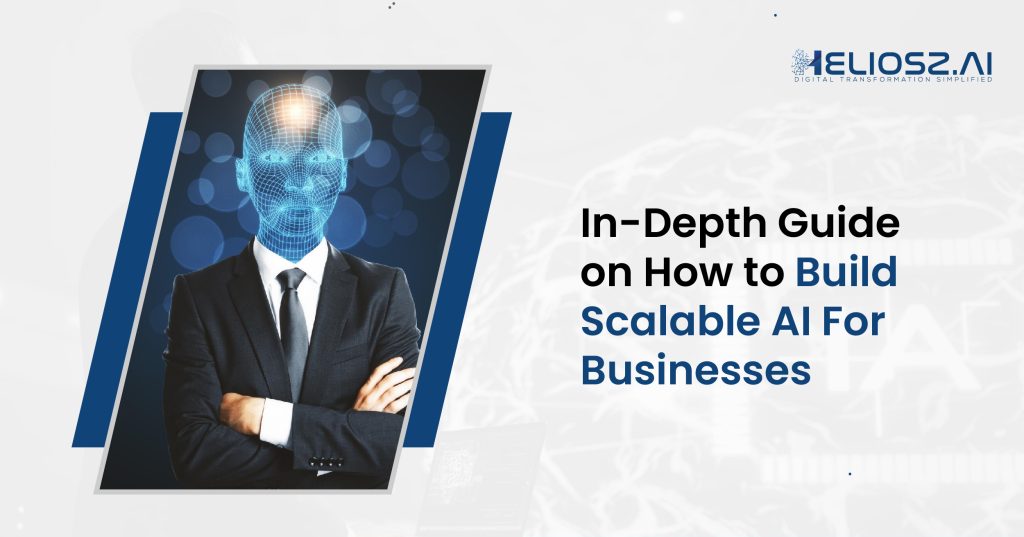
Organizations operate highly in this fast-paced, data-driven world, relying increasingly on AI to optimize their operations. However, growing and evolving organizations increase their needs and create new challenges from the surface. Scaling AI solutions may work with a small-scale operation, but it might struggle to keep up with the requirements of more significant, complex business environments. So, it’s essential to have scalable AI for the futureproofing of AI implementations. Such systems need to be scalable in a way that they can respond well to the growing demands without having to be redone altogether. If not implemented, such businesses are likely to lag behind competitors who can grow and cope with the demands. Let’s discuss Scalable AI in this informative article.
Are you hunting for an understanding of scalable AI? If yes, then this detailed guide is the only one you need to know!
What is Scalable AI?
Scalable AI refers to artificial intelligence systems that can deal with increasing volumes of data, increasing complexity, and increasing demand from users efficiently without compromising performance. It ensures the seamless adaptation of any business AI capabilities to growth in a manner that is strictly proportional to the growing nature of the business as it incurs more and more workloads, new tasks, or new environments. Scalable AI refers to the ability to process large amounts of data, support complex algorithms, and maintain peak performance over a variety of operational conditions. Scalable AI efficiency in solutions means they are nimble and resilient to cost with the ability to scale up capacity efficiently and sustainably as the business requires it to.
Core Technologies Behind Scalable AI
1. Machine Learning (ML) and Deep Learning (DL)
Machine learning and deep learning constitute the base for scalable AI systems. ML allows algorithms to find an apparent pattern in data to make predictions while deep learning, being an advanced term connected with ML, uses deep neural networks to process complex, vast amounts of data. It is very suitable for image recognition and natural language processing activities.
2. Cloud Computing
Cloud platforms offer flexible, on-demand computing capabilities so that businesses may scale their AI systems with minimal investment in hardware. Services from AWS, Google Cloud, and Azure feature infrastructure for big dataset storage, model training, and AI application deployment.
3. Big Data Technologies
Useful tools for processing large data volumes are big data tools like Flink, AWS Glue, or Google BigQuery. Such technologies enable scalable AI systems to process large amounts of information at high speeds. Therefore, such models can be learned from huge datasets. All these can eventually lead to valuable insights from AI models.
4. Edge Computing
It can process close to the source, reducing latency associated with real-time decisions. For instance, edge computing applications that have a pressing need for instant responses would be more critical for latency-sensitive applications than others. Some possible examples of how edge computing is applied with autonomous vehicles and IoT devices, and how it can scale AI systems quite more efficiently in remote or distributed environments.
5. Containerization and Microservices
Scalable AI comes because of containerization (e.g., Docker) and microservices, which break applications down into smaller, independent services. This allows them to be deployed easily and scaled through many environments, as components can grow or shrink to meet the need without impacting the whole system.
Steps to Build Scalable AI for Your Business
A strategic approach to building scalable AI for your business ensures that the system can keep up with the pace of your organization’s needs while enhancing performance and still being reliable. Here are the core steps towards building scalable AI:
Step 1- Data Collection and Preparation
The foundation of any AI system is high-quality, relevant data. Businesses must start by collecting data from various sources such as customer interactions, transaction records, and operational systems. All these data require cleansing, normalization, and proper structuring to get it ready for an AI-processing purpose. It includes removing inconsistencies, handling missing values, and transforming the data formats to best suit AI model training. The better the quality and richness of the data, the better it is expected to perform with an AI system.
Step 2- Building the Right AI Models
Choosing the right type of AI model is critical for scalability. The choice depends on the type of problem and business needs. For example, supervised learning uses labeled data to train models for specific tasks such as classification or regression, while unsupervised learning is used to discover patterns in unlabeled data. Reinforcement learning, on the other hand, involves systems that learn through trial and error to optimize decision-making, as seen in applications like robotics or autonomous driving. An appropriate model must be capable of handling large datasets efficiently and adapting to evolving business requirements with minimal retraining.
Step 3- Infrastructure Set-up to be Scalable
Scalable AI requires heavy infrastructure. Therefore, the company needs to choose to go for cloud options, on-premises solutions, or both. Cloud platforms such as AWS, Google Cloud, and Microsoft Azure ensure the scale for large-scale AI applications. There are computing resources, storage resources, and dedicated AI tools in these platforms that will help businesses scale up their AI systems very smoothly with a hike in data volume and complexity.
Step 4- Scaling Models Training and Testing
Scalability training of AI models requires the use of distributed computing resources, for example, cloud GPUs or parallel processing, to deal with large datasets efficiently. At the same time, it is necessary to ensure that the models do not overfit the data and generalize well for data they haven’t seen before. To test scalability, there is also stress testing under heavy loads such that the business model, even with growth or additional data, still performs well.
Step 5- Scalable Deployment Strategies for AI Systems
After training and testing the model, it is time to deploy. Scalable deployment strategies involve practices of continuous integration and continuous deployment that enable models to update automatically as new data arrives. Containerization by Docker also proves helpful in making scalable deployments as AI models can be packaged into containers with no issues and can run across different environments. Furthermore, a strategy of deployment should be able to support an ability to scale up or down on short notice depending on demand.
Implementing these steps can help businesses achieve effective and scalable AI systems that adapt to changing needs and expand their operations with time.
Building Scalable AI Strategies with Heliosz.AI
Heliosz.AI enables businesses to develop comprehensive, all-in-one solutions so that the integration of AI into the organization runs as seamlessly as possible. Our teams, comprising experts, collaborate with your organization to design and build AI systems that grow with your business, ensuring efficiency, flexibility, and success over the long term. With an intimate understanding of both AI technology and business processes, we tailor a solution that’s not only scalable but also aligned with your strategic objectives. From data preparation to model building and onward to its deployment along with ongoing optimization, Heliosz.AI offers end-to-end support, making it easier for businesses to unlock full AI potential and stay ahead in the competitive market.
Conclusion: Future-Proof Your Business with Scalable AI Solutions
Scalable AI is the future of business for companies willing to invest in adaptive, future-forward solutions. The more a company focuses on scalability, the less chance it has of getting stuck on demand-related issues and being unable to make AI adjust. A variety of options comes with AI building, both robust infrastructure and modular design, as well as even cloud-native tools, that impact its ability to grow sustainably. Scalable AI readiness prepares companies to meet the current business objectives and paves the way for a dynamic future where AI will be an even more integral part of such an organization