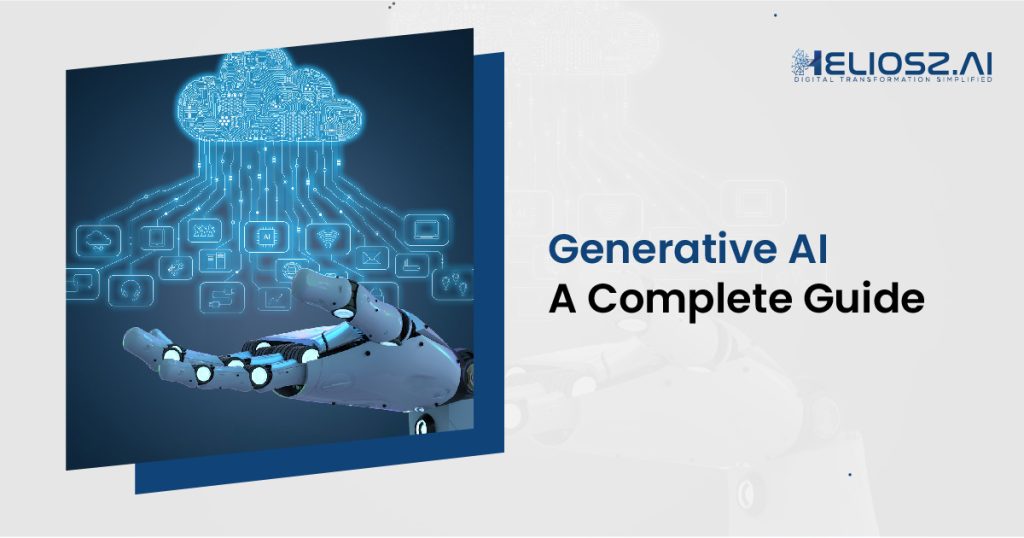
AI has undergone many revolutionary changes, and one of the most innovative frontiers is that of generative AI. This is an area of AI that revolutionizes how machines think, create content, and solve problems while interacting with humans. It includes such applications as developing lifelike images, generating music, and simulating human conversations, to name a few, that extend the limits of what can be done by technology. This guide explores the intricacies of generative AI, from how it works to its models and applications and its impact on industries worldwide.
What is Generative AI?
Generative AI represents a type of artificial intelligence that is aimed at generating new content through patterns learned from existing data. As opposed to traditional AI systems that operate according to previously designed rules for accomplishing certain tasks, generative AI models generate text, images, videos, music, and other types of content that are quite indistinguishable from human-created content.
At its core, generative AI is about creativity: allowing machines to emulate the creative process. Good examples are the text-generating GPT from OpenAI, DALL-E generating images, and music composition, like AIVA.
How Generative AI Works
1. Collection and Preprocessing
Collection and cleaning of large datasets based on desired output, like text images, audio, etc. And then it standardizes data for training.
2. Design of Model Architecture
Based on task selected model architecture. The GAN is popularly known for images, transformers for text, and VAEs for data variations.
3. Training the Model
The model learns patterns from data, computes errors, and adjusts parameters through iterative training, which requires high computational resources.
4. Pattern and Feature Learning
The AI identifies patterns, relationships, and structures in the training data such as grammar in text or shapes in images to guide its outputs.
5. Content Generation
The trained models use learned patterns to create new outputs, such as text, images, or music, often starting from prompts or random inputs.
6. Fine-Tuning and Validation
The model is fine-tuned with task-specific data and validated to ensure quality and accuracy, addressing any issues found during testing.
7. Deployment and Real-World Use
After validation, the model is deployed for practical use. Continuous monitoring is done to ensure the optimal performance of the model and updates are done based on the need.
Understanding Generative AI Models and Its Types
Generative AI models represent powerful tools, capable of producing new realistic data based on learning by patterns of already existing data. Hereunder, descriptions of the major types of generative AI models are given:
1. GANs (Generative Adversarial Networks)
GANs are made up of two networks: a generator that produces data and a discriminator that assesses it. The generator improves over time by trying to fool the discriminator, thus producing realistic outputs. GANs are widely used for generating lifelike images, videos, and even deepfake content, making them ideal for creative applications.
2. Variational Autoencoders (VAEs)
VAEs compress input data with an encoder and then reconstruct it with a decoder. They introduce randomness, which allows for smooth variations in output. VAEs are good for tasks like modifying facial features in images, generating new designs, and detecting anomalies in data.
3. Transformers
Transformers process data with self-attention, which helps them understand long-range dependencies in a dataset. Therefore, they are very good at text generation, such as GPT models, and flexible for other types of data, like images and music. Transformers form the backbone of modern natural language processing.
4. Diffusion Models
Diffusion models transform random noise into sharp data through iterative refinement. These models are much more successful in generating good-quality visuals than GANs and are used for producing and editing images.
5. Recurrent Neural Networks (RNNs) and LSTMs
RNNs, and more recently LSTMs, process inputs serially but do have some memory about previous steps of sequential data generation. LSTMs over the traditional RNN will handle longer dependencies, useful for text or music creation, although the application of transformer has somewhat changed the mainstream.
6. Autoregressive Models
Autoregressive models generate data sequentially, with each element based on the previous one. This ensures coherence and logical flow, making them highly effective for text generation and sequential data tasks like pixel-by-pixel image creation.
What are Some Popular Generative AI Applications?
Generative AI revolutionized almost all sectors by being able to create content, solve problems, and innovate processes. Some of its main applications follow:
1. Content Generation
The creation of digital content is transformed through generative AI. With GPT-4, Jasper, and Copy.ai, for instance, articles, marketing copy, product descriptions, and even social media posts can be generated. Time is saved, and high-quality content is ensured both for the creator and the business.
2. Image and Video Generation
These models, including DALL-E, Stable Diffusion, and MidJourney, create absolutely amazing visual things, ranging from artistic art pieces to completely hyper-realistic images. Generative AI also underpins deepfake technology: replacing one’s face or even synthesizing speech is the heart of entertainment and media in use.
3. Music Composition
Generative AI tools such as AIVA and OpenAI’s MuseNet can generate original music across genres. These models help musicians and content creators produce soundtracks, jingles, or even full-length compositions with minimal effort.
4. Game Development
Generative AI could be designing characters, environments, even entire storylines for games. This technology automates the creation of textures and animations, reducing the actual development time and cost. The idea is to improve creative work on game design itself.
5. Healthcare and Medicine
Generative AI in health care generates synthetic medical data, accelerates drug discovery, and simulates protein structures. These help with improvements in diagnostics and breakthroughs in personalized medicine.
6. Customer Service
Through generative AI models like ChatGPT, chatbots and virtual assistants can simulate human-like conversations. They offer 24/7 support, efficiently handle customer queries, and personalize interactions to provide better user experiences.
7. Education and Training
Generative AI helps in the development of interactive learning materials, personalized study plans, and simulations. It also automates grading and feedback, which enhances teaching and learning for educators and students.
Advantages and Benefits of Generative AI
1. Creativity and Innovation
Generative AI opens up new levels of creativity, developing original content from quite realistic images to music and text. This opens new avenues for artists, writers, designers, to experiment with novel ideas, generate inspiration and find new ways to push the creative boundaries. For example, tools like DALL-E or AIVA can be used to create unique artwork or musical compositions that never would have existed otherwise.
2. Efficiency and Automation
Generative AI automates tedious and time-consuming repetitive tasks, such as content generation, data analysis, or design prototyping. Such handling of tasks enables professionals to concentrate on more strategic or creative work at a more strategic level, thus achieving higher productivity. Businesses also utilize AI-driven tools such as Jasper and Canva for the quick and efficient creation of marketing materials.
3. Cost Cutting
Generative AI reduces operational costs for numerous companies by streamlining workflows and minimizing time for tasks. For example, in gaming and animation businesses, AI generates assets such as characters or textures, which require less manual labor without compromising quality. Therefore, innovation becomes more accessible to smaller companies and new startups.
4. Personalization
Generative AI, in areas such as marketing and e-commerce, allows for hyper-personalization. AI systems analyze user data to tailor recommendations, advertisements, and learning plans according to individual preferences, thus increasing user engagement and satisfaction. This is also good for businesses and consumers alike because of better outcomes.
5. Rapid Prototyping and Simulation
Generative AI will accelerate product design innovation as it enables the rapid prototyping and simulation of solutions. This allows engineers and designers to test ideas, point out flaws, and further refine their designs without extended physical testing. This is a very critical area in automotive and aerospace.
6. Improved Decision-making
Generative AI helps analyze huge datasets and generate insights for better decision-making. For example, in finance, AI models help generate scenarios for risk assessment, while in health care, they assist in diagnosing diseases or identifying treatment plans based on patient data.
7. Scalability
Generative AI scales well to meet the demands of large projects. For example, a business can generate hundreds of pieces of content, such as product descriptions or advertisements, in a fraction of the time it would take a human team. This scalability is particularly valuable for organizations aiming to grow rapidly.
8. Increased Accessibility
Generative AI will therefore make advanced tools and resources not only accessible but also user-friendly for non-experts, for instance, to write professional-quality content on the scale of ChatGPT or Canva.
9. Knowledge and Skills Gap Bridging
Generative AI helps both individuals and organizations in places where such expertise may not exist. At the educational level, it prepares customized learning material, whereas at the business level, it automates difficult tasks, such as coding or data analysis, hence bridging skill gaps and further enhancing capabilities.
How is Generative AI Changing Business?
1. Customer Support and Chatbots
This generative AI is improving the service provided to customers with the help of AI-powered chatbots and virtual assistants, completing inquiries from customers in a conversation for quick and accurate responses 24/7. These AI systems understand natural language, enabling businesses to arrive at faster resolutions, reduce operational costs, and enhance customer satisfaction. They also have a learning curve from past interactions, which improves their capability to answer complex queries.
2. Financial Modeling and Risk Analysis
Generative AI models also aid businesses in the finance industry by generating simulations of market trend patterns, investment strategies, and risk scenarios. Data tools like DataRobot and Kensho support financial analysts in forecasting through past data to help analyze data and make better decisions on financial performance. Such AI-led systems can process vast sums of data quickly to look at trends, assess risks, and present the best investment plans or portfolio management options for improving financial performance.
3. Talent Recruitment and HR
Generative AI affects the HR industry by making talent recruitment automated and optimizing hiring. Tools like HireVue use generative models in analyzing resumes, conducting video interviews, and even deciding whether a candidate is appropriate for a certain role based on their responses. This helps HR departments identify top talent faster, reduce human bias in hiring decisions, and streamline recruitment workflows to make the process more efficient and cost-effective.
4. Supply Chain Optimization
This will allow organizations to maximize supply chain activities. AI systems analyze historical data and market trends, along with other external factors such as weather conditions or geopolitical events that may disrupt the supply chain. This will help predict disruptions within the supply chain and manage inventories better. For example, IBM’s Watson Supply Chain platform leverages AI for businesses to predict their delivery delays, adjust purchase strategies to balance delivery times, ultimately save costs, and improve operational efficiency.
5. Automatic Legal Document
Generative AI changes the face of the legal industry, automating document creation for legal documents, contracts, and compliance reports. Technologies like DoNotPay and LawGeex use AI to draft and review legal documents that comply with regulations, saving time for lawyers in routine paperwork. The automation of more complex legal matters allows law teams to focus on matters of more complexity while at the same time improving efficiency and cutting costs.
6. Manufacturing and Production Efficiency
The power of generative AI for manufacturing optimization in production scheduling and resource and process designs was seen, for instance, through Siemens’ MindSphere with AI in predictive maintenance scheduling, resulting in reduced time in equipment idle hours and enhancing productivity. AI-driven optimization helps in producing goods quicker, with less waste and more efficiency, thereby making production costs lower and enhancing profitability.
7. Personalized Customer Experiences
Businesses are now using generative AI to deliver unique experiences to customers. For example, e-commerce platforms such as Amazon or Netflix use recommendations that are AI-generated according to the preferences of a user, their purchase history, and browsing history. The systems are self-improving with machine learning so that businesses can offer specific promotions, personalized product suggestions, and dynamic pricing strategies. This personalized approach increases customer engagement and loyalty and subsequently sales.
Bottomline
Generative AI is not merely a technological advancement but, rather, a paradigm change that allows machines to engage with humans in creating and innovating to solve more complex problems. With businesses and individuals unlocking their potential, generative AI will unlock possibilities, creativity, and efficiency for industries. Key to such benefits will be the adoption of this technology responsibly and ethically to maximize the benefits that it brings while also addressing possible challenges.