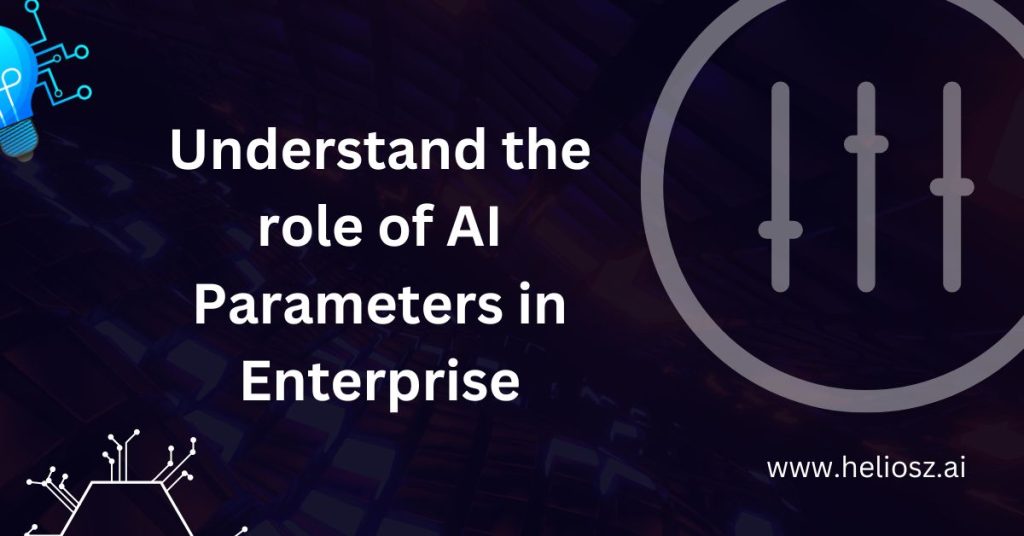
Artificial intelligence (AI) has become an undeniable presence in enterprise settings such as intelligent automation to streamline workflows and machine learning algorithms to optimize marketing campaigns. Optimal performance and efficacy are the expected outcomes from enterprise AI applications and solutions to justify the investment in training AI models. This goal can only be achieved by focusing on a strategic approach to defining AI parameters systematically. This is the crucial element that plays a significant role in defining the capabilities and limitations of AI in enterprise settings.
Table of Contents
- What are AI parameters?
- Types of AI Parameters
- Significance of AI Parameters in Enterprise Applications
- Challenges of Fine-Tuning AI Parameters
Let’s dig into the intricacies of AI parameters in the enterprise AI ecosystem through this informative article.
What are AI parameters?
AI parameters encompass a broad spectrum of variables that govern and control the outcomes of artificial intelligence models. These are the key elements that determine the behavior and performance of AI models. Consider a vast network of interconnected nodes that represent a piece of data. The nodes are connected by many connectors and the strength of connectors between these nodes – the parameters – determines the overall efficiency of the network. In conclusion, parameters are the building blocks of intelligence within an AI model. The greater the number of parameters, the more potent the AI models become!
Types of AI Parameters
1. Hyperparameters:
This type of AI parameter controls the architecture and configuration of AI models which are configuration settings external to the model. These parameters dictate the learning rate and number of hidden layers in a neural network.
2. Model Parameters:
Model parameters represent the internal variables of AI models. These are optimized during the training process to reduce the prediction errors and increase the AI model performance.
3. Training Parameters:
This category of AI parameters (also known as optimization parameters) includes aspects like batch size, optimization algorithms, etc. Training parameters influence the training dynamics and convergence of AI models. It also regulates convergence speed, computational efficiency, and the ability of the model to generalize unseen data.
Significance of AI Parameters in Enterprise Applications
AI parameters serve as the linchpin for reaping the full potential of AI technologies in enterprises. The operational efficiency and effectiveness of AI systems in enterprises are optimized by well-defined AI parameters. Below are the major key points and benefits of AI parameters in enterprise AI applications.
1. Optimizing Model Performance
The optimization of model performance is crucially represented by the level of fine-tuning conducted for AI parameters. Enterprises can train AI systems to deliver precise predictions and actionable insights by meticulous adjustments to parameters. These parameters include learning rates, regularization techniques, and network architectures. Optimization of model performance drives enterprises towards staying ahead of the curve in data-driven modern business environments. This will enable informed decision-making and initiate strategic operations.
2. Improving Efficiency in AI parameters
The strategic and systematic tuning of AI parameters is the prominent key to fostering computational efficiency and enhancing resource utilization in enterprises. Judicious tweaking of AI parameters related to data processing, feature selection, and model optimization will lead to streamlined computational processes. This will significantly save time and overhead costs. Along with operational efficiency, this practice will enable considerable cost savings and empower enterprises to invest in the areas where more strategic focus is demanded.
3. Enhancing Accuracy
Careful selection and smart optimization of AI parameters ensure that enterprise AI models are capable of delivering accurate and robust results. Enterprises can fine-tune their AI models to achieve superior performance metrics and strengthen the reliability and accountability of AI-driven decision-making processes.
4. Streamlining Operations
AI parameters play a significant role in streamlining various operations across enterprise workflows. Automation of mundane tasks, optimizing supply chain logistics, efficient resource allocation, upgrading marketing activities, and many other important business operations. By embedding AI-driven automation and optimization into critical operational functions such as supply chain management, customer relationship management, and inventory forecasting, enterprises can achieve greater operational resilience and responsiveness in an increasingly dynamic business landscape.
Challenges of Fine-Tuning AI Parameters
There are a multitude of challenges associated with fine-tuning AI parameters in enterprises. Organizations must investigate and tackle these challenges to achieve optimal model performance with utmost accuracy.
1. Data Quality and Bias
The quality of training data determines the success of AI parameters fine-tuning in AI application settings. Any little bit of inaccuracies in training data will get amplified in AI models and it will lead to delivering unfavorable outcomes. Hence it is crucial to conscientiously choose and gather data used for fine-tuning to reduce the transfer of errors throughout the model performance.
2. Overfitting and Underfitting
Finding an equilibrium and balance between overfitting and underfitting is one of the prominent challenges in fine-tuning AI parameters. Overfitting refers to the situation where the AI models remember the training data too much and generate results accordingly. This situation will lead to the incapability of the model to deliver generalized results on unseen data. In contrast, underfitting denotes the performance scenario where the model generates too simplistic or general results without capturing the underlying patterns in the data.
3. Computational Resources
Fine-tuning AI parameters is a complex procedure and technique that requires significant computational resources. Organizations with constraints on budget and infrastructure often find this an expensive deal and overly challenging. Optimizing parameters will involve running numerous iterations of training algorithms on large datasets and this will add to the complexity of AI fine-tuning processes.
4. Domain-Specific Considerations
AI parameters should be optimized based on the domain requirements. Different industries and applications have unique metrics and requirements. Implementing AI models to perform domain-specific tasks needs domain-specific knowledge and expertise.
5. Hyperparameter Search Space
Hyperparameters are considered an important factor when it comes to governing the overall efficacy of AI models. The search space for hyperparameters in AI models is really broad and complicated. It is challenging to identify the optimal configuration. Grid search, random search, and Bayesian optimization are the popular techniques to narrow down the search space. Still, hyperparameter tuning is a time-consuming process that requires deep expertise and experimentation.
6. Catastrophic Forgetting
In some cases, models will forget what they learned during pre-training when they are trained with new data. This is known as catastrophic forgetting, and it is a major setback during fine-tuning AI parameters. Enterprises need to focus on techniques like elastic weight consolidation to train models to remember the knowledge gained from previous tasks.
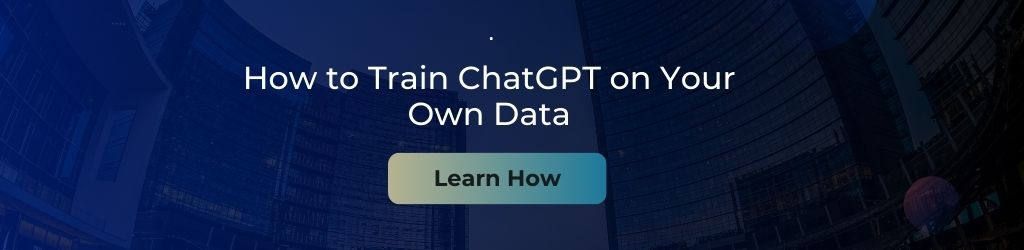
Frequently Asked Questions About AI Parameters
What does the number of parameters mean in AI?
In AI, the parameter count represents the factors a model acquires during training. In essence, they explain the complexity of the model and its capability to understand and depict patterns in data. Increased parameters often lead to a more complex model that can capture intricate relationships, but they also come with higher computational requirements and the risk of overfitting.
What is the difference between parameters and hyperparameters?
Parameters are variables inside a model that are acquired through training, while hyperparameters are external settings that impact the training and performance of a model but are not learned from data.
How do AI parameters work?
AI parameters are numeric values that AI systems adjust during training to enhance performance. They essentially govern how the AI understands and reacts to information. Developers can refine the AI’s actions by modifying these settings, enabling it to improve its decision-making skills and achieve its set targets more effectively.
Why Heliosz.AI is Your Best AI Consulting Partner?
Implementing AI models to automate complex business operations cannot be achieved with top-notch expertise levels and meticulous planning. The ecosystem of data, artificial intelligence models, AI parameters, and all other huge data pools are as complicated as seeming almost unachievable. But with the right AI consulting partner like Heliosz.AI, all these AI optimizations in enterprises are effortless endeavors.
Here’s why Heliosz.ai stands out as your premier AI consulting partner:
- Heliosz.ai boasts a team of seasoned data scientists, machine learning engineers, and AI experts with extensive experience in optimizing AI models across various industries.
- We take a tailored approach to every engagement, carefully analyzing your unique business requirements, data characteristics, and domain-specific challenges to develop customized solutions that drive tangible results.
- Access to cutting-edge AI technologies and tools. We leverage the latest innovations to maximize the performance and efficiency of your AI systems.
- With a proven track record of success, Heliosz.ai has helped numerous enterprises across industries achieve remarkable results through AI optimization.
- We work closely with your internal teams, leveraging your domain expertise and business insights to co-create solutions that align with your strategic objectives and deliver tangible business value.
- From initial consultation to ongoing support and maintenance, Heliosz.ai provides comprehensive end-to-end support throughout your AI journey.
At Heliosz.ai, excellence is not just a goal – it’s a commitment. We are dedicated to delivering best-in-class AI solutions that exceed your expectations and drive measurable impact for your organization.
Contact us today to learn more about how we can empower your organization to thrive in the age of AI.